As a clinician, I have been keen to apply the principles of evidence-based medicine, informed by evidence from systematic reviews and the specific needs of each of my patients. However, in my attempts to practise evidence-based medicine, I have often had to confront two frustrations. First, many clinical decisions involve more than two possible interventions, yet systematic reviews are usually based only on comparisons in pairs of interventions. Second, the comparisons researched do not cover all the relevant interventions.
Many others have experienced these frustrations. For example, after assessing 18 trials testing 24 interventions for children with acute pyelonephritis, John Ioannidis (2006) asked: “How do we make sense of this complex network and guess the best choice(s)?”
It was in 2009 that I first became aware of an approach to addressing quandaries resulting from such complex networks. The solution involves combining direct and indirect treatment comparisons using evidence from randomised trials to produce a synthesis linking data from all the interventions in a ‘network’. In doing this, each trial shares at least one direct comparison with another trial. Analysis of the network – network meta-analysis (NMA) – could then produce a ranking of the likelihood of each of the interventions being the most effective.
Network meta-analysis as part of the development of research synthesis
Network meta-analysis is a method of research synthesis, so any history of this specific method must acknowledge that it is just one part of the overall history of research synthesis. That wider history has been described by others (Chalmers et al. 2002; Clarke 2015). This article focuses on the origins and development of this method of synthesis, which has also been described as a ‘mixed treatment comparison’ or ‘multiple treatments comparison’ (Lee 2014).
The concept of network meta-analysis
Network meta-analysis is an extension of traditional, pairwise meta-analysis, but three main advantages are claimed for network meta-analysis. Firstly, it allows more than two interventions to be compared simultaneously; secondly, interventions can be compared even if they have not been directly compared in trials; and thirdly, it increases precision of the estimate of effect size by ‘borrowing strength’ (Higgins and Whitehead 1996) if the key assumptions described below are valid.
When seeking to compare the effects of interventions A, B and C, if there are trials of A versus B and B versus C, but not of A versus C, an estimate of the relative effects of A compared to C can be made by indirect comparison using the results of the trials of A versus B and B versus C. This indirect comparison uses the results of meta-analysis of all trials in each direct comparison, as explained by Bucher et al (1997), to combine, in a single synthesis, all the available direct comparisons and all the indirect comparisons that can be estimated between the selected interventions.
A network diagram can be drawn illustrating the direct comparisons between A, B and C. In more complex networks, multiple combinations of direct comparisons are used to estimate the same indirect comparison, which are then incorporated in the synthesis. These networks might have more than three interventions and have varying complexity of geometry. Among the simplest is a star, in which the intervention at the centre is the common comparator to all the other interventions. More complex forms of network geometry, when there are common comparators for only a few of a more diverse range of interventions, involve multiple conjoined loops and sidearms.
Figure 1 shows an example of a network diagram from a network meta-analysis comparing six interventions, A to F. The solid lines show where trial results of direct comparisons exist. In this example, there is no single common comparator for all the interventions, but each intervention shares at least one comparator with another intervention in the network. The thickness of each connecting line represents the number of trials between the pair of interventions at either end of the line and the size of each node (letter within a circle) represents the number of participants receiving the intervention. Some network diagrams also include the actual numbers of trials, participants or both.
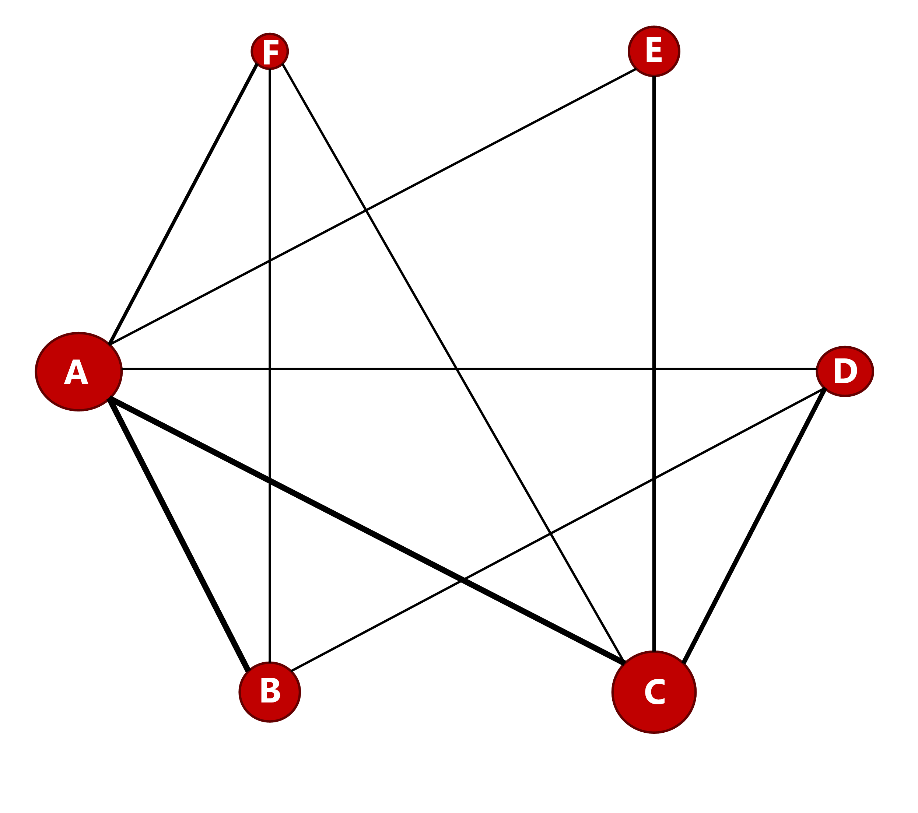
Figure 1. Network diagram example
Transitivity and consistency are two key assumptions for these analyses. Assessing the validity of these requires input of both clinical and methodological expertise. Transitivity is the assumption that effect modifiers (the clinical and methodological characteristics that can affect the outcome) are similar in each direct comparison involving the same intervention (Cipriani et al. 2013). It cannot be assessed statistically but requires critical interpretation of the effect modifiers in the trials whose results are considered for synthesis. Before a network meta-analysis is conducted, those trials must be assessed for significant differences in their populations, interventions, outcomes, methodological features and reporting (Efthimiou et al. 2016). It is also important to note that even placebo response has been found to vary over time, which might affect the transitivity assumption when placebo is a common comparator (Julious and Wang 2008).
Consistency is an extension of transitivity. It is the assumption of agreement between the results of direct and indirect comparisons for each pair of interventions. This can be assessed statistically but only when there are both direct and indirect comparisons of one or more pairs of interventions within a network, known as ‘closed loops. The assumption of transitivity needs to be reconsidered if inconsistency is detected. If inconsistency is not detected statistically, however, that does not automatically validate the transitivity assumption (Cipriani et al. 2013).
Origins and evolution of methods for network meta-analysis
In 1989, Eddy described the ‘Confidence Profile Method’ (Eddy 1989), and the Eddy, Hasselblad and Shachter publication (Eddy et al. 1990) in the following year, “A Bayesian Method for Synthesising Evidence”, described:
“a collection of meta-analysis techniques based on Bayesian methods for interpreting, adjusting, and combining evidence to estimate parameters and outcomes important to the assessment of health technologies.”
These techniques were collectively called the ‘Confidence Profile Method’ (CPM) and the article explained indirect comparison with the following example:
“The approach is to use the available evidence to derive probability distributions for the various pairs that have been directly compared. A distribution for the relative effects of other pairs can then be calculated by a series of convolutions. The concept is illustrated by calculating the difference between the test scores of Tom and Bill from knowledge of the differences in scores between Tom and George, and George and Bill.”
Practical application of the methodology was supported by software to conduct the synthesis. Eddy initially used SOFT*PRO, but the commonest software in published systematic reviews reporting network meta-analysis (Lee 2014) is WinBUGS (initially BUGS) (Lunn et al. 2000). This uses Bayesian methods, and such methods are predominant in both software use and methodology developments in network meta-analysis, but frequentist approaches and software are also used (Jansen et al. 2011; Hoaglin et al. 2001).
A series of methodology publications through the 1990s built on the CPM approach, including notably those by Smith, Spiegelhalter and Thomas (1995) and by Higgins and Whitehead (1996). Higgins and Whitehead wrote about borrowing strength from external trials in a meta-analysis. They argued that
“Many meta-analysis papers include data from three or more treatments, but only consider pairwise comparisons of, say treatment A with control and treatment B with control. There would seem to be little reason not to combine all treatments into one analysis.”
They used the BUGS software to combine the results of trials comparing the effects of beta-blockers versus sclerotherapy, beta-blockers versus control and sclerotherapy versus control on preventing cirrhosis-related bleeding. Higgins’ and Whitehead’s 1996 paper was described later by Salanti and Schmid (2012) as:
“the first to articulate that relative effects of different treatments can be jointly estimated in a single meta-analysis model to improve power. This landmark paper introduced the basis for the methodology which, now extended and refined, is increasingly known as network meta-analysis.”
In 2002, Lumley published ‘Network meta-analysis for indirect treatment comparisons’ in which he
“presented methods of estimating treatment differences between treatments that have not been directly compared in a randomized trial, and, more importantly, methods of estimating the uncertainty in these differences.” (Lumley 2002)
Lumley acknowledged the limitation of his methods, which were restricted to each trial only having two intervention groups
“Meta-analyses with large numbers of multi-armed trials present difficulties for network meta-analysis, and extensions to handle multi-armed trials correctly should be investigated.”
Ades subsequently described methods to encompass multi-arm trials and multiple outcomes. In his 2003 article (Ades 2003), he stated
“The aim of nearly all meta-analysis has been to summarize evidence comparing one or sometimes more treatments. Usually only a single outcome is examined, and if there is more than one outcome these are explored in separate meta-analyses, rather than simultaneously. This paper concerns the possibility of combining information from different studies on different, but structurally related, outcomes, and using the data to construct a single model which expresses the relationships between the different kinds of data.”
The following year, together with Lu, Ades published ‘Combination of direct and indirect evidence in mixed treatment comparisons’ (Lu and Ades 2004), which was the most frequently cited origin of current network meta-analysis methodology in a survey of published network meta-analyses in 2014 (Lee 2014). Lu and Ades extended the model proposed by Smith, Spiegelhalter and Thomas (1995) to encompass trials with more than two intervention groups.
A review of the methods for network meta-analysis, with particular emphasis on the issue of inconsistency between direct and indirect evidence, was published in 2008 by Salanti et al. (2008). They explained that inconsistency in estimates of intervention effects obtained from direct and indirect comparisons may indicate diversity, bias or a combination of both, and they described modelling to test for consistency. Their review considered potential sources of inconsistency, including genuine diversity in the characteristics of included trials, selection bias, study quality and sponsorship bias. It stresses the importance of planning in advance for investigation of inconsistency, because clinical and epidemiological assessment of inconsistency may be difficult because of factors such as reporting deficiencies or lack of sufficient studies for some comparisons. Salanti et al. also highlighted that attention to the geometry (the overall pattern of comparisons amongst interventions) and the asymmetry of networks (the extent to which specific comparisons of interventions are represented more heavily than others in the number of included trials or participants) can be used to inform the design of the new trials that would most usefully add to the overall network.
A review of network meta-analysis methods, published in 2016 by Efthimiou et al. (2016), summarised newer publications on the use of network meta-analysis methods. This included various models for performing network meta-analysis, statistical methods for assessing inconsistency, software options, investigating sources of potential bias and reporting results.
The use of individual participant data (IPD) in meta-analyses has many advantages over the use of aggregate data, including improving the quantity and quality of data, which has resulted in it being considered ‘the gold standard in evidence synthesis’ (Debray et al. 2018). The use of this approach, initially using traditional, pairwise meta-analysis methods, increased between the early 1990s and 2008 to around 50 publications per year (Riley et al. 2010). The number of systematic reviews using IPD was found to be 10 to 22 per year with no discernible growth trend in the years leading up to 2015 (Simmonds et al. 2015). Gao et al. found that the first IPD using network meta-analysis methods was published in 2007 and that 21 IPDs using network meta-analysis methods had been published by June 2019 (Gao et al. 2020). There are limitations as well as advantages to use of IPD. Guidance has been published on the best use of IPD meta-analysis generally (Tierney et al. 2015) and specifically on the use of network meta-analysis methods with IPD (Debray et al. 2018).
Multiple outcomes multivariate meta-analysis (MOMA) is another approach to meta-analysis that has been increasing in recent years (Riley at al. 2017). Relevant studies that might be considered for synthesis may not report the same outcomes, which could result in their exclusion from traditional meta-analyses, but MOMA allows for inclusion where outcomes can be regarded as highly correlated. Guidance on conducting this type of synthesis using network meta-analysis methods has been published in recent years (Riley et al. 2017; Achana et al. 2014; Efthimiou et al. 2014), including the use of IPD (Riley et al. 2015).
Interest has developed recently in creating and maintaining continuously updated meta-analyses using network meta-analysis methods (Vandvik et al. 2016) and a major project of this kind for COVID-19-related interventions began in 2020 (Boutron et al. 2020).
Approaches to conducting network meta-analysis
A simple meta-regression approach can be used for network meta-analysis if there is no multi-arm trial in the network (Cochrane Collaboration 2019). However, if the network includes multi-arm trials, other methods are more appropriate. Bayesian methods have been used most frequently (Nikolakopoulu et al. 2014), partly because this approach can most naturally produce estimates of ranking probabilities for the interventions being compared (to give the probability that each intervention is most effective through to least effective) (Neupane et al. 2014); but frequentist methods to approximate ranking have also been described (White 2011). The hierarchical model approach is detailed by Lu and Ades (2004) and by Salanti (2008).
An alternative approach is multi-variate meta-analysis, which can be conducted using Bayesian (Mavrides and Salanti 2013) or frequentist methods (White et al. 2012). A further approach, based on graph-theoretical methods, has been described by Rucker (2012).
The frequentist approach assumes that the intervention effect has a true value with a confidence interval which defines the range within which the true value would fall with a minimum probability, usually 95%. The Bayesian approach assumes that the intervention effect has a fixed value but within a probability distribution based on a ‘prior’, which might be a value chosen from existing evidence or might be a ‘best guess’. The credible interval results of a Bayesian meta-analysis provide the probability of the range of values within which the fixed value lies, given the data, and this range is the ‘posterior’ that includes 95% of the probability.
Use of network meta-analyses in published systematic reviews
In 1999, Dominici et al. used Bayesian methods and data from 46 trials of treatments to prevent migraine headache, to produce a ranking of treatments. They stated their aims as follows:
“In this article we present a meta-analysis of these 46 trials with the goal of synthesizing existing evidence about which treatments are most effective and of quantifying the remaining uncertainty about treatment effectiveness. We hope that the results and methods will be useful in supporting clinical treatment decisions and will help guide the planning of new trials. The critical statistical aspects of this goal are the estimation of treatment effects on a common scale and the relative ranking of treatments, both within classes and overall. This requires indirect comparisons among treatments that may never have been tested together in the same trial.”
Dominici et al. used data collected by another team working on a systematic review. The first published report of a network meta-analysis as part of a systematic review conducted by the authors, appears to have been that reported by Psaty et al. in 2003. Their explanation for selecting this method to study treatments for high blood pressure summarises well the commonest problem in using only pairwise meta-analysis to inform clinical decision making:
“The clinical trials in hypertension have provided a patchwork of evidence about the health benefits of antihypertensive agents. Some trials used placebo or untreated controls, and others used active-treatment comparison groups. Among the latter, the choice of treatment and comparison therapies has varied from one trial to the next. Several approaches to the synthesis of these complex data are possible. The Blood Pressure Trialists, for instance, conducted a prospective series of mini-meta-analyses, but this method left many “unresolved issues” due to multiple comparisons and low power. In this study, we used a new technique, called network meta-analysis, to synthesize the available evidence from placebo-controlled and comparative trials in a single meta-analysis.”
Subsequent uses of network meta-analysis were initially slow to appear in the healthcare literature. Edwards et al. (2009) identified only seven published systematic reviews reporting network meta-analysis up to July 2007. Publications rose to an estimated 90 to 100 published in 2012 (Lee 2014) and an estimated 180 to 200 in 2018 (Lee 2020), with a total of more than 1000 now available in the literature. The increasing trend of publication of network meta-analyses is illustrated in Figure 2. The solid line indicates the number of publications for each year up to 2012 identified by a review (Lee 2014); the dashed line extends this to 2020 using the results of Medline searches for each year. This trajectory of increasing publications is similar to that for publications reporting use of traditional, pairwise meta-analysis between 1980 and 2000 (Lee et al. 2001).
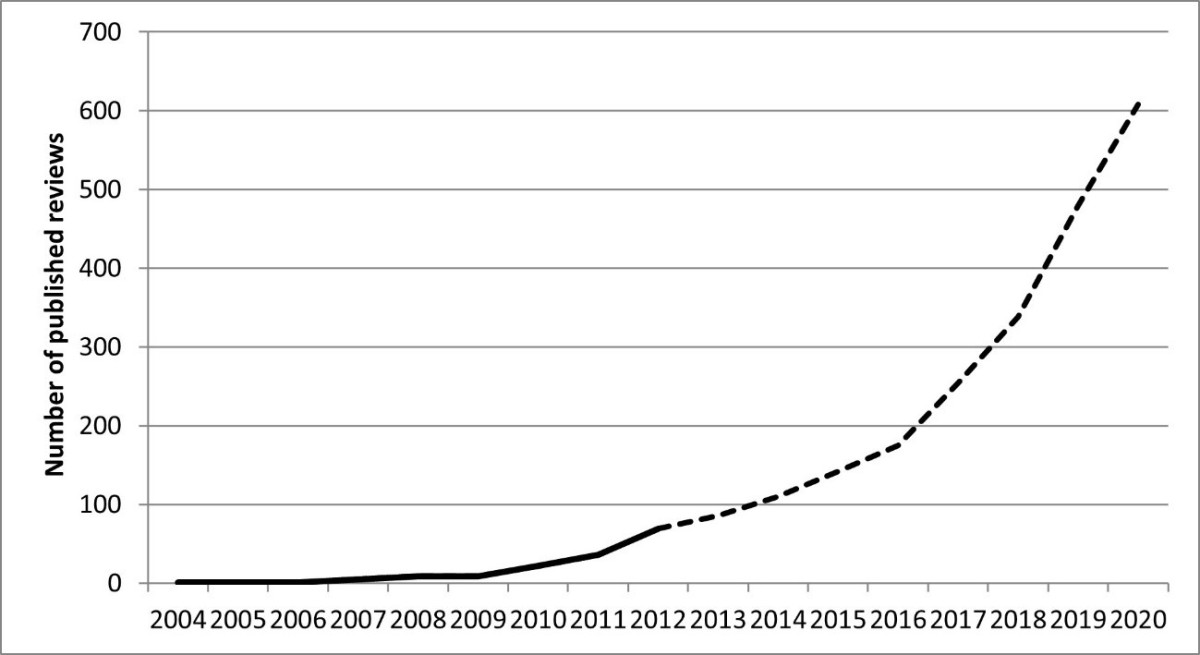
Figure 2. Estimate for the number of published network meta-analyses to 2020
The most significant impact of evidence synthesis on health care is likely to come from the use of the evidence generated by these research projects in national clinical guidelines. A review of NICE clinical guidelines published or updated in 2015 and 2016 found that they made extensive use of meta-analysis to identify evidence to support their recommendations (Lee 2020). network meta-analysis methods were used far less often than traditional, pairwise meta-analysis but were used or considered for nearly one quarter of the guidelines reviewed, showing that evidence produced using network meta-analysis methods is influencing recommendations for the UK National Health Service.
Guidance relating to conduct and reporting of network meta-analysis
Efforts to improve the quality of reporting of systematic reviews have been based on defining and promoting internationally recognised standards. There is evidence that publication of such reporting standards results in improved quality of reporting, based on comparisons before and after the publication of both the QUOROM Statement (Moher et al. 1999) and the PRISMA Statement (Liberati et al. 2009), and that reporting to PRISMA Statement standards is strongly associated with higher study quality, as assessed by a widely used Critical Appraisal Tool (Tunis et al. 2013). Panic et al. (2013) found that endorsement of the PRISMA Statement by journals in their instructions for authors was associated with improved quality of reporting, regardless of whether the authors declared that they had followed the Statement and was associated with higher study quality.
When the first standards for reporting systematic reviews and meta-analyses were published in 1999 in the QUORUM Statement, the concept of network meta-analysis was still in its infancy. However, by the time of the PRISMA Statement in 2009, network meta-analysis warranted a mention as a form of meta-analysis that combined direct and indirect comparisons. However, the PRISMA 2009 statement did not make recommendations relating to reporting that addressed the specifics of network meta-analysis methodology. For standards for conducting systematic reviews, it directed readers to the guidance published by the Cochrane Collaboration (2011) and the Centre for Reviews and Dissemination (2009), both of which contained very limited guidance on the use of network meta-analysis methodology in systematic reviews. Reporting standards for use of network meta-analysis methods were subsequently published as a PRISMA Extension Statement in 2015 (Hutton et al. 2015).
The Methods Guide 2008 Update of the National Institute for Health and Care Excellence (NICE) included a section on network meta-analysis for the first time and the NICE Decision Support Unit’s Evidence Synthesis TSD series (2002), published initially in 2011, expanded on that guidance. Also in 2011, the International Society for Pharmacoeconomics and Outcomes Research (ISPOR) published reports on the interpretation and conduct of network meta-analysis (Jansen et al.2011; Hoaglin et al. 2011). Ades (2011) and observed that this “seems to represent the first position statement from an academic body on these methods”
The Cochrane Comparing Multiple Interventions Methods Group (CMIMG) was established in 2010 and has since produced guidance on the use of network meta-analysis methods in Cochrane Reviews and promoted training in using these methods. Version 6 of the Cochrane Handbook for Systematic Reviews of Interventions, published in 2019, contained ‘a major new core chapter’ addressing for the first time network meta-analysis within the Handbook. This guidance emphasises that network meta-analysis is ‘more statistically complex than a standard meta-analysis’, consequently, ‘close collaboration’ between a statistician with expertise in network meta-analysis methods and those with expertise in the clinical content area is essential in the design and conduct of a review to ensure that studies selected for inclusion in network meta-analysis fulfil the assumptions of transitivity and consistency (Chaimani et al. 2019).
Critical appraisal of network meta-analysis
Critical appraisal involves assessing the report of a study for methodological quality and any likelihood of bias and considering whether these might affect the validity of the reported results. A 2018 review of published critical appraisal tools for systematic reviews (Lee 2020) found that none of the most widely used critical appraisal tools (CATs) for systematic reviews contained content specifically relevant to appraising research synthesis using network meta-analysis methodology. Three tools had been published which include content that is relevant to appraising the use of network meta-analysis methods (Jansen et al. 2011; Ades et al. 2012; Ortego et al. 2014). These three tools, however, might not be suitable for end-users without specialist statistical knowledge of network meta-analysis methodology, so there is still potentially a role for a new critical appraisal tool to support generalist end-users. A tool has been constructed using the CASP format (CASP 2017), which might form the basis for further development (Lee 2020).
Other approaches have been developed to assess confidence in the evidence produced using network meta-analysis methods. For example, in 2014, the GRADE Working Group reported guidance for establishing the quality of treatment effect estimates obtained from network meta-analysis (Puhan et al. 2014). Their approach involved rating the quality of each direct and indirect effect estimate for each pairwise comparison within the network meta-analysis and then rating the network meta-analysis effect estimate for each pairwise comparison. In 2018, the GRADE Working Group recommended modifications to their 2014 guidance with a view to making the process more efficient, acknowledging that the original approach, ‘may appear onerous in networks with many interventions’ (Brignardello-Petersen et al. 2018).
In 2014, Salanti et al. published a modification of the GRADE guidance, in particular, drawing a distinction between rating the effect estimates for each pairwise comparison and rating the ranking of all the interventions within a network (Salanti et al. 2014). More recently, in 2020, Salanti and others published a new approach: Confidence in Network Meta-Analysis (CINeMA) (Nikolakopoulu et al. 2020). They stated that uptake of the earlier 2014 GRADE system and that reported by Salanti and others in the same year had been limited by ‘the complexity of the methods and the lack of suitable software’.
CINeMA is also based on the GRADE framework but instead of considering direct and indirect evidence for each pairwise comparison separately, it considers the impact of every study in the network. A web-based application makes it easy to apply to even large networks.
A further approach, ‘threshold analysis’, has been developed specifically to assess confidence in network meta-analysis results used in guideline development (Phillippo et al. 2019). The authors argue that their approach is needed because GRADE approaches do not assess the influence of the network meta-analysis evidence on a resulting recommendation:
‘Threshold analysis quantifies precisely how much the evidence could change (for any reason, such as potential biases, or simply sampling variation) before the recommendation changes, and what the revised recommendation would be. If it is judged that the evidence could not plausibly change by more than this amount, then the recommendation is considered robust; otherwise, it is sensitive to plausible changes in the evidence.’
These alternative approaches are primarily intended to be used by authors of systematic reviews and guideline developers (to rate confidence in an estimate of effect or confidence that such an estimate adequately supports a specific recommendation), but they are relevant to understanding by those undertaking critical appraisal and can be used to complement use of a critical appraisal tool.
Conclusions
Network Meta-Analysis is a relatively new form of evidence synthesis and evolution of the methodology is continuing. As outlined by Salanti (2012), the introduction of network meta-analysis faced similar scepticism to that raised originally about traditional, pairwise meta-analysis. However, there has been gradually wider uptake of the method by researchers and use of the resulting evidence by decision makers in health and social care. The Cochrane review with the largest number of included studies is a network meta-analysis of 585 randomised trials of drugs to prevent post-operative nausea and vomiting (Weibel et al. 2021). Consensus guidance relating to the conduct and reporting of network meta-analysis is now widely available.
The use of network meta-analysis methods can overcome some limitations of traditional, pairwise meta-analysis. The design and conduct of network meta-analyses requires multidisciplinary input from expert methodologists and clinical topic experts. Further research is needed to clarify whether end-users who do not have specialist statistical knowledge can assess the quality and validity of evidence produced in systematic reviews using network meta-analysis methods, even with a critical appraisal tool optimised for such studies.
This James Lind Library article has been republished in the Journal of the Royal Society of Medicine 2022;115:313-321. Print PDF
References
Achana FA, Cooper NJ, Bujkiewicz S, Hubbard SJ, Kendrick D, Jones DR, Sutton AJ (2014). Network meta-analysis of multiple outcome measures accounting for borrowing of information across outcomes. BMC Medical Research Methodology 14:92. DOI: 10.1186/1471-2288-14-92.
Ades AE (2003). A chain of evidence with mixed comparisons: models for multi-parameter synthesis and consistency of evidence. Statistics in Medicine 22:2995-3016. DOI: 10.1002/sim.1566.
Ades AE (2011). ISPOR states its position on network meta-analysis. Value in Health 14:414-416. DOI: 10.1016/j.jval.2011.05.001.
Ades AE, Caldwell DM, Reken S, Welton NJ, Sutton AJ, Dias S (2012). NICE DSU Technical Support Document 7: Evidence Synthesis Of Treatment Efficacy In Decision Making: A Reviewer’s Checklist https://www.ncbi.nlm.nih.gov/books/NBK395872/ (accessed 27 May 2022).
Boutron I, Chaimani A, Meerpohl JJ, Hróbjartsson A, Devane D, Rada G, Tovey D, Grasselli G, Ravaud P; COVID-NMA Consortium (2020). The COVID-NMA Project: Building an Evidence Ecosystem for the COVID-19 Pandemic. Annals of Internal Medicine 173:1015-7. DOI: 10.7326/M20-5261.
Brignardello-Petersen R, Bonner A, Alexander PE, Siemieniuk RA, Furukawa TA, Rochwerg B, Hazlewood GS, Alhazzani W, Mustafa RA, Murad MH, Puhan MA, Schünemann HJ, Guyatt GH; GRADE Working Group (2018). Advances in the GRADE approach to rate the certainty in estimates from a network meta-analysis. Journal of Clinical Epidemiology 93:36-44. DOI: 10.1016/j.jclinepi.2017.10.005.
Bucher HC, Guyatt GH, Griffith LE, Walter SD (1997). The results of direct and indirect treatment comparisons in meta-analysis of randomized controlled trials. Journal of Clinical Epidemiology 50: 683-691. DOI: 10.1016/s0895-4356(97)00049-8.
Centre for Reviews and Dissemination (2009). Systematic reviews: CRD’s guidance for undertaking reviews in health care. CRD, University of York. https://www.york.ac.uk/inst/crd/index_guidance.htm (accessed 27 May 2022).
Chaimani A, Caldwell DM, Li T, Higgins JPT, Salanti G (2019). Chapter 11: Undertaking network meta-analyses. In: Higgins JPT, Thomas J, Chandler J, et al. (eds) Cochrane Handbook for Systematic Reviews of Interventions Version 60. https://www.training.cochrane.org/handbook (accessed 27 May 2022).
Chalmers I, Hedges LV, Cooper H (2002). A brief history of research synthesis. Evaluation and the Health Professions 25:12-37.
CINeMA Confidence in Network Meta-Analysis. https://cinema.ispm.unibe.ch/ (accessed 27 May 2022).
Cipriani A, Higgins JP, Geddes JR, Salanti G (2013). Conceptual and technical challenges in network meta-analysis. Annals of Internal Medicine 159: 130-137. DOI: 10.7326/0003-4819-159-2-201307160-00008.
Clarke M (2015). History of evidence synthesis to assess treatment effects: personal reflections on something that is very much alive. JLL Bulletin: Commentaries on the history of treatment evaluation, http://www.jameslindlibrary.org/articles/history-of-evidence-synthesis-to-assess-treatment-effects-personal-reflections-on-something-that-is-very-much-alive/
The Cochrane Collaboration (2011). Cochrane Handbook for Systematic Reviews of Interventions, Version 5.1.0 [updated March 2011]. In: Higgins JPT, Green S (eds): https://training.cochrane.org/handbook/archive/v5.1/ (accessed 27 May 2022).
Cochrane Collaboration (2019). In: Higgins JPT, Thomas J, Chandler J, et al. (eds). Cochrane Handbook for Systematic Reviews of Interventions Version 6.0 [updated July 2019]. https://www.training.cochrane.org/handbook (accessed 27 May 2022).
Critical Appraisal Skills Programme (2017). CASP Systematic Review Checklist. https://casp-uk.net/images/checklist/documents/CASP-Systematic-Review-Checklist/CASP-Systematic-Review-Checklist_2018.pdf (accessed 29 November 2022).
Debray TP, Schuit E, Efthimiou O, Reitsma JB, Ioannidis JP, Salanti G, Moons KG; GetReal Workpackage (2018). An overview of methods for network meta-analysis using individual participant data: when do benefits arise? Statistical Methods in Medical Research 27:1351-1364. DOI: 10.1177/0962280216660741.
Dominici F, Parmigiani G, Wolpert RL, Hasselblad V (1999). Meta-analysis of migraine headache treatments: combining information from heterogeneous designs. Journal of the American Statistical Association 94:16-28. DOI: 10.1080/01621459.1999.10473815.
Eddy DM (1989). The confidence profile method: a Bayesian method for assessing health technologies. Operations Research 37:210-228. DOI: 10.1287/opre.37.2.210.
Eddy DM, Hasselblad V, Shachter R (1990). A Bayesian method for synthesizing evidence. The Confidence Profile Method. International Journal of Technology Assessment in Health Care 6(1):31-55. DOI: 10.1017/s0266462300008928.
Edwards SJ, Clarke MJ, Wordsworth S, Borrill J (2009). Indirect comparisons of treatments based on systematic reviews of randomised controlled trials. International Journal of Clinical Practice 63:841-854. DOI: 10.1111/j.1742-1241.2009.02072.x.
Efthimiou O, Mavridis D, Cipriani A, Leucht S, Bagos P, Salanti G (2014). An approach for modelling multiple correlated outcomes in a network of interventions using odds ratios. Statistics in Medicine 33:2275-2287. DOI: 10.1002/sim.6117.
Efthimiou O, Debray TP, van Valkenhoef G, Trelle S, Panayidou K, Moons KG, Reitsma JB, Shang A, Salanti G; GetReal Methods Review Group (2016). GetReal in network meta-analysis: a review of the methodology. Research Synthesis Methods 7:236-263. DOI: 10.1002/jrsm.1195.
Gao Y, Shi S, Li M, Luo X, Liu M, Yang K, Zhang J, Song F, Tian J (2020). Statistical analyses and quality of individual participant data network meta-analyses were suboptimal: a cross-sectional study. BMC Medicine 18:120. DOI: 10.1186/s12916-020-01591-0.
Higgins JP, Whitehead A (1996). Borrowing strength from external trials in a meta-analysis. Statistics in Medicine 15:2733-2749. DOI: 10.1002/(SICI)1097-0258(19961230)15:24<2733::AID-SIM562>3.0.CO;2-0.
Hoaglin DC, Hawkins N, Jansen JP, Scott DA, Itzler R, Cappelleri JC, Boersma C, Thompson D, Larholt KM, Diaz M, Barrett A (2011). Conducting indirect-treatment-comparison and network-meta-analysis studies: report of the ISPOR Task Force on Indirect Treatment Comparisons Good Research Practices: part 2. Value in Health 14:429-437. DOI: 10.1016/j.jval.2011.01.011.
Hutton B, Salanti G, Caldwell DM, Chaimani A, Schmid CH, Cameron C, Ioannidis JP, Straus S, Thorlund K, Jansen JP, Mulrow C, Catalá-López F, Gøtzsche PC, Dickersin K, Boutron I, Altman DG, Moher D (2015). The PRISMA Extension statement for reporting of systematic reviews incorporating network meta-analyses of health care interventions: Checklist and explanations. Annals of Internal Medicine 162:777-784. DOI: 10.7326/M14-2385.
Ioannidis JP (2006). Indirect comparisons: the mesh and mess of clinical trials. Lancet 368:1470-1472. DOI: 10.1016/S0140-6736(06)69615-3.
Jansen JP, Fleurence R, Devine B, Itzler R, Barrett A, Hawkins N, Lee K, Boersma C, Annemans L, Cappelleri JC (2011). Interpreting indirect treatment comparisons and network meta-analysis for health-care decision making: report of the ISPOR Task Force on Indirect Treatment Comparisons Good Research Practices: part 1. Value in Health 14:417-428. DOI: 10.1016/j.jval.2011.04.002.
Julious SA, Wang S-J (2008). How Biased Are Indirect Comparisons, Particularly When Comparisons Are Made Over Time in Controlled Trials? Drug Information Journal 42:625-633. DOI: 10.1177/009286150804200610.
Lee AW (2014). Review of mixed treatment comparisons in published systematic reviews shows marked increase since 2009. J Clin Epidemiol 67:138-143. DOI: 10.1016/j.jclinepi.2013.07.014.
Lee A (2020). Developing critical appraisal of systematic reviews reporting network meta-analysis. DPhil, University of Oxford. https://ora.ox.ac.uk/objects/uuid:b9b878bd-fc04-4317-9df5-781930224f6b (accessed on 27 May 2022).
Lee WL, Bausell RB, Berman BM (2001). The growth of health-related meta-analyses published from 1980 to 2000. Evaluation and the Health Professions 24:327-335.
Liberati A, Altman DG, Tetzlaff J, Mulrow C, Gøtzsche PC, Ioannidis JP, Clarke M, Devereaux PJ, Kleijnen J, Moher D (2009). The PRISMA statement for reporting systematic reviews and meta-analyses of studies that evaluate healthcare interventions: explanation and elaboration. BMJ 339: b2700. DOI: 10.1136/bmj.b2700.
Lu G, Ades AE (2004). Combination of direct and indirect evidence in mixed treatment comparisons. Statistics in Medicine 23: 3105-3124. DOI: 10.1002/sim.1875.
Lumley T (2002). Network meta-analysis for indirect treatment comparisons. Statistics in Medicine 21:2313-2324. DOI: 10.1002/sim.1201.
Lunn DJ, Thomas A, Best N, Spiegelhalter D (2000). WinBUGS — a Bayesian modelling framework: concepts, structure, and extensibility. Statistics and Computing 10:325-337. DOI: 10.1023/a:1008929526011.
Mavridis D, Salanti G (2013). A practical introduction to multivariate meta-analysis. Statistical Methods in Medical Research 22: 133-158. DOI: 10.1177/0962280211432219.
Moher D, Cook DJ, Eastwood S, Olkin I, Rennie D, Stroup DF (1999). Improving the quality of reports of meta-analyses of randomised controlled trials: the QUOROM statement. Quality of Reporting of Meta-analyses. Lancet 354: 896-1900. DOI: 10.1016/s0140-6736(99)04149-5.
National Institute for Health and Clinical Excellence (2008). Guide to the methods of technology appraisal. https://www.nice.org.uk/process/pmg9/resources/guide-to-the-methods-of-technology-appraisal-2013-pdf-2007975843781 (accessed 29 November 2022).
Neupane B, Richer D, Bonner AJ, Kibret T, Beyene J (2014). Network meta-analysis using R: a review of currently available automated packages. PLoS One 9:e115065. DOI: 10.1371/journal.pone.0115065.
NICE Decision Support Unit (DSU). Evidence Synthesis TSD series, http://www.nicedsu.org.uk/Evidence-Synthesis-TSD-series(2391675).htm (accessed 27 May 2022).
Nikolakopoulou A, Chaimani A, Veroniki AA, Vasiliadis HS, Schmid CH, Salanti G (2014). Characteristics of networks of interventions: a description of a database of 186 published networks. PLoS One 9:e86754. DOI: 10.1371/journal.pone.0086754.
Nikolakopoulou A, Higgins JPT, Papakonstantinou T, Chaimani A, Del Giovane C, Egger M, Salanti G (2020). CINeMA: An approach for assessing confidence in the results of a network meta-analysis. PLoS Medicine 2020; 17: e1003082. DOI: 10.1371/journal.pmed.1003082.
Ortega A, Fraga MD, Alegre-del-Rey EJ, Puigventós-Latorre F, Porta A, Ventayol P, Tenias JM, Hawkins NS, Caldwell DM (2014). A checklist for critical appraisal of indirect comparisons. Int J Clin Pract 68:1181-1189. DOI: 10.1111/ijcp.12487.
Panic N, Leoncini E, de Belvis G, Ricciardi W, Boccia S (2013). Evaluation of the endorsement of the preferred reporting items for systematic reviews and meta-analysis (PRISMA) statement on the quality of published systematic review and meta-analyses. PLoS One 8:e83138. DOI: 10.1371/journal.pone.0083138.
Phillippo DM, Dias S, Welton NJ, Caldwell DM, Taske N, Ades AE (2019). Threshold analysis as an alternative to GRADE for assessing confidence in guideline recommendations based on network meta-analyses. Annals of Internal Medicine 170:538-546. DOI: 10.7326/M18-3542.
Psaty BN, Lumley T, Furberg CD, Schellenbaum G, Pahor M, Alderman MH, Weiss NS (2003). Health outcomes associated with various antihypertensive therapies used as first-line agents – a network meta-analysis. JAMA 289:2534-2544. DOI: 10.1001/jama.289.19.2534.
Puhan MA, Schünemann HJ, Murad MH, Li T, Brignardello-Petersen R, Singh JA, Kessels AG, Guyatt GH; GRADE Working Group (2014). A GRADE Working Group approach for rating the quality of treatment effect estimates from network meta-analysis. BMJ 349:g5630. DOI: 10.1136/bmj.g5630.
Riley RD, Lambert PC, Abo-Zaid G. (2010). Meta-analysis of individual participant data: rationale, conduct, and reporting. BMJ 340:c221. DOI: 10.1136/bmj.c221.
Riley RD, Price MJ, Jackson D, Wardle M, Gueyffier F, Wang J, Staessen JA, White IR (2015). Multivariate meta-analysis using individual participant data. Research Synthesis Methods 6:157-174. DOI: 10.1002/jrsm.1129.
Riley RD, Jackson D, Salanti G, Burke DL, Price M, Kirkham J, White IR (2017). Multivariate and network meta-analysis of multiple outcomes and multiple treatments: rationale, concepts, and examples. BMJ 358:j3932. DOI: 10.1136/bmj.j3932.
Rucker G (2012). Network meta-analysis, electrical networks and graph theory. Res Synth Methods 3:312-324. DOI: 10.1002/jrsm.1058.
Salanti G, Higgins JP, Ades AE, Ioannidis JP (2008). Evaluation of networks of randomized trials. Statistical Methods in Medical Research 17:279-301. DOI: 10.1177/0962280207080643.
Salanti G (2012). Indirect and mixed-treatment comparison, network, or multiple-treatments meta-analysis: many names, many benefits, many concerns for the next generation evidence synthesis tool. Research Synthesis Methods 3:80-97. DOI: 10.1002/jrsm.1037.
Salanti G, Schmid CH (2012). Research Synthesis Methods special issue on network meta-analysis: introduction from the editors. Research Synthesis Methods 3:69-70. DOI: 10.1002/jrsm.1050.
Salanti G, Del Giovane C, Chaimani A, Caldwell DM, Higgins JP (2014). Evaluating the quality of evidence from a network meta-analysis. PLoS One 9:e99682. DOI: 10.1371/journal.pone.0099682.
Simmonds M, Stewart G, Stewart L (2015). A decade of individual participant data meta-analyses: A review of current practice. Contemporary Clinical Trials 45:76-83. DOI: 10.1016/j.cct.2015.06.012.
Smith TC, Spiegelhalter DJ, Thomas A (1995). Bayesian approaches to random-effects meta-analysis: a comparative study. Statistics in Medicine 14:2685-2699. DOI: 10.1002/sim.4780142408.
Tierney JF, Vale C, Riley R, Smith CT, Stewart L, Clarke M, Rovers M (2015). Individual Participant Data (IPD) Meta-analyses of randomised controlled trials: guidance on their use. PLoS Medicine 12:e1001855. DOI: 10.1371/journal.pmed.1001855.
Tunis AS, McInnes MD, Hanna R, Esmail K (2013). Association of study quality with completeness of reporting: have completeness of reporting and quality of systematic reviews and meta-analyses in major radiology journals changed since publication of the PRISMA statement? Radiology 269:413-426. DOI: 10.1148/radiol.13130273.
Vandvik PO, Brignardello-Petersen R, Guyatt GH. (2016). Living cumulative network meta-analysis to reduce waste in research: A paradigmatic shift for systematic reviews? BMC Medicine 14:59. DOI: 10.1186/s12916-016-0596-4.
Weibel S, Pace NL, Schaefer MS, Raj D, Schlesinger T, Meybohm P, Kienbaum P, Eberhart LHJ, Kranke P (2021). Drugs for preventing postoperative nausea and vomiting in adults after general anesthesia: An abridged Cochrane network meta-analysis. Journal of Evidence Based Medicine 14:188-197. DOI: 10.1111/jebm.12429.
White IR (2011). Multivariate random-effects meta-regression: Updates to Mvmeta. The Stata Journal 11:255-270. DOI: 10.1177/1536867×1101100206.
White IR, Barrett JK, Jackson D, Higgins JP (2012). Consistency and inconsistency in network meta-analysis: model estimation using multivariate meta-regression. Research Synthesis Methods 3:111-125. DOI: 10.1002/jrsm.1045.